Protein sequencing with tandem mass spectrometry is a powerful technique used to identify and characterize proteins. However, the success of this technique relies heavily on proper data analysis and interpretation. In order to obtain accurate and reliable results, it is important to follow best practices in analyzing the mass spectrometry data. This includes proper preprocessing of raw data, selection of appropriate algorithms for database searching and peptide identification, and thorough validation of the results. By adhering to these best practices, researchers can ensure that their protein sequencing experiments yield meaningful insights into the composition and function of complex biological samples.
Ensuring the Accuracy and Reliability of Protein Sequencing Data Obtained Through Tandem Mass Spectrometry
To ensure the accuracy and reliability of protein sequencing data obtained through tandem mass spectrometry, several steps can be taken. Firstly, it is important to use high-quality instrumentation and follow standardized protocols for sample preparation and analysis. This includes proper calibration of the mass spectrometer, optimization of fragmentation methods, and careful data processing to minimize errors. Additionally, using multiple technical replicates and incorporating quality control measures such as internal standards or spike-in controls can help validate the results. It is also crucial to compare the obtained data with known protein sequences from databases and perform rigorous statistical analysis to confidently identify and quantify the proteins present in the sample. Regularly updating and maintaining the database used for protein identification can further enhance the accuracy and reliability of the results.
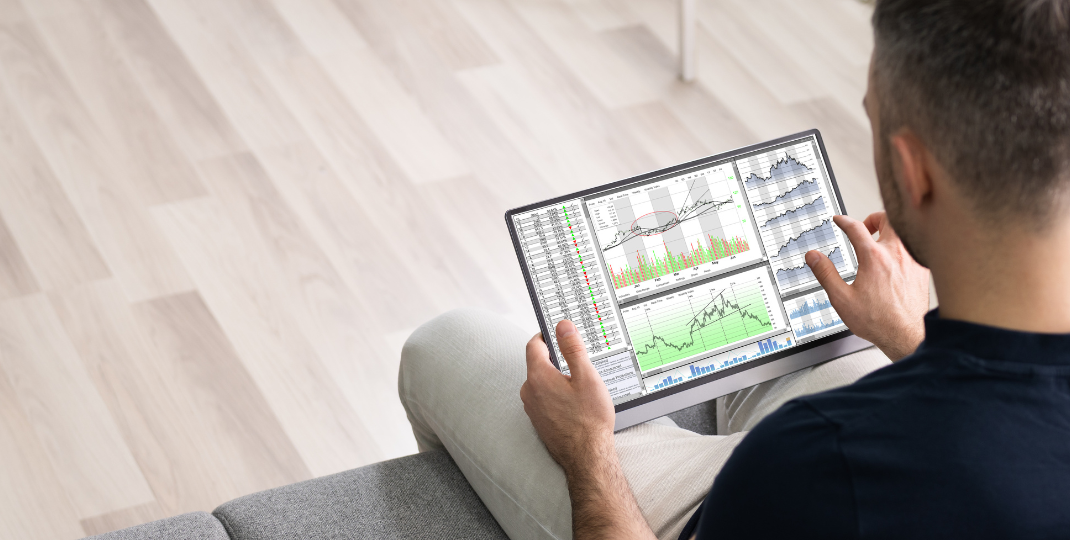
What are the most effective strategies for identifying and quantifying proteins in complex samples using tandem mass spectrometry?
The most effective strategies for identifying and quantifying proteins in complex samples using tandem mass spectrometry involve several key steps. First, sample preparation methods such as protein extraction, digestion, and fractionation are crucial for separating and purifying the proteins of interest. Next, high-resolution liquid chromatography coupled with tandem mass spectrometry (LC-MS/MS) is used to separate and ionize the peptides generated from the digested proteins. Database searching algorithms are then employed to match the acquired fragmentation spectra to known protein sequences, allowing for protein identification. Lastly, quantification can be achieved through label-free or isotopic labeling methods, where the abundance of specific peptides or proteins is measured based on their MS signal intensity or ratio compared to a reference sample. Overall, careful experimental design, data processing, and validation are essential for accurate and reliable protein identification and quantification using tandem mass spectrometry.
How do we handle missing or incomplete data in protein sequencing results obtained from tandem mass spectrometry?
Missing or incomplete data in protein sequencing results obtained from tandem mass spectrometry can be handled through various strategies such as imputation methods, which involve estimating the missing values based on known data patterns. Another approach is to perform sensitivity analyses by omitting the incomplete data and assessing the impact on the overall results. Additionally, researchers can consider using multiple imputation techniques to generate multiple plausible values for the missing data points and incorporate uncertainty into the analysis. It is important to carefully document any assumptions or methods used to handle missing data in order to ensure the validity and reliability of the protein sequencing results.
What are the potential biases and limitations of data analysis and interpretation in protein sequencing with tandem mass spectrometry?
Potential biases and limitations in data analysis and interpretation of protein sequencing with tandem mass spectrometry include sample contamination, instrument errors, database incompleteness, and software limitations. Sample contamination can lead to false identification of proteins, while instrument errors such as noise or signal drift can affect the accuracy of peak detection and quantification. Database incompleteness may result in failure to identify certain proteins, and software limitations can introduce bias in data processing and interpretation. Additionally, the complexity of the data generated by tandem mass spectrometry can make it challenging to accurately interpret and analyze results, leading to potential errors in protein identification and quantification.
How can we effectively integrate different data analysis tools and software for comprehensive protein sequencing analysis?
Integrating different data analysis tools and software for comprehensive protein sequencing analysis can be achieved by first identifying the specific needs and goals of the analysis. Next, it is important to evaluate the compatibility and interoperability of the different tools and software to ensure seamless integration. Utilizing data management platforms that support multiple formats and allow for easy data transfer between tools can also streamline the process. Additionally, establishing a standardized workflow and communication protocol among team members using the various tools can help ensure consistency and efficiency in the analysis process. Regular training and updates on the latest advancements in the field of proteomics can further enhance the integration of different tools and software for comprehensive protein sequencing analysis.
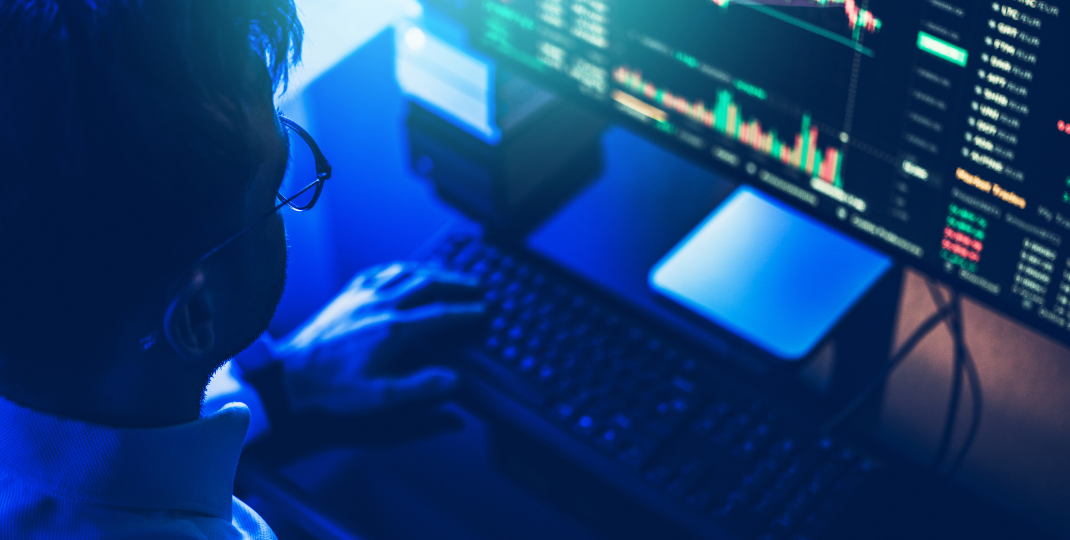
What are the best methods for validating and confirming protein identifications and modifications in tandem mass spectrometry data?
The best methods for validating and confirming protein identifications and modifications in tandem mass spectrometry data typically involve a combination of different approaches. These can include using multiple search engines to increase confidence in identifications, performing database searches with stringent criteria, conducting manual verification of spectra, and utilizing targeted or parallel reaction monitoring assays for specific validation of modified peptides. Additionally, the use of isotopic labeling techniques, such as SILAC or iTRAQ, can help confirm protein identifications by comparing ratios of labeled peptides across different conditions. Overall, a comprehensive validation strategy that incorporates various techniques is essential for ensuring the accuracy and reliability of protein identification and modification data obtained from tandem mass spectrometry experiments.
How do we account for variability and noise in protein sequencing data when interpreting results from tandem mass spectrometry experiments?
Variability and noise in protein sequencing data from tandem mass spectrometry experiments can be accounted for by using statistical methods to identify and filter out unreliable data points. This can involve performing replicate experiments to assess the consistency of results, applying signal-to-noise ratio thresholds to exclude low-quality spectra, and employing algorithms that correct for systematic biases in the data. Additionally, bioinformatics tools can be used to compare experimental results against databases of known proteins to validate identifications and assign confidence scores to the findings. By taking these steps to account for variability and noise, researchers can ensure the accuracy and reliability of their interpretations of protein sequencing data from tandem mass spectrometry experiments.
What are the current challenges and future directions in improving data analysis and interpretation practices for protein sequencing with tandem mass spectrometry?
The current challenges in improving data analysis and interpretation practices for protein sequencing with tandem mass spectrometry include the complexity and volume of data generated, the need for robust algorithms to accurately identify and quantify proteins, the lack of standardized protocols for data processing, and the difficulty in integrating multiple datasets for comprehensive analysis. Future directions in this field involve developing advanced machine learning and artificial intelligence techniques to enhance data processing efficiency and accuracy, establishing standardized pipelines and quality control measures for data analysis, incorporating multi-omics approaches for more comprehensive characterization of complex biological systems, and expanding the use of open-access databases and resources for data sharing and collaboration among researchers. Additionally, there is a growing emphasis on the integration of quantitative proteomics with other omics technologies to provide a more holistic view of cellular processes and disease mechanisms.
The importance of best practices in data analysis and interpretation for protein sequencing with tandem mass spectrometry
In conclusion, the best practices for data analysis and interpretation in protein sequencing with tandem mass spectrometry involve thorough pre-processing of raw data, careful selection of suitable software and algorithms, rigorous quality control measures, and validation of results through independent experiments or bioinformatics tools. It is crucial to have a clear understanding of the experimental design, biological context, and limitations of the technology to ensure accurate and reliable interpretation of the data. Collaboration with experts in the field and adherence to standardized protocols can also greatly enhance the quality and reproducibility of protein sequencing studies using tandem mass spectrometry. By following these best practices, researchers can confidently draw meaningful conclusions and make significant contributions to the field of proteomics.
