Advancements in software tools and bioinformatics have greatly improved peptide identification by mass spectrometry in recent years. These advancements have allowed researchers to more accurately and efficiently analyze complex data generated from mass spectrometry experiments, leading to a deeper understanding of biological processes at the molecular level. By utilizing advanced algorithms and databases, scientists are now able to identify peptides with higher confidence levels, leading to more reliable results and insights into protein structure and function. This progress has opened up new possibilities for proteomics research and has paved the way for further discoveries in the field of bioinformatics.
Integrating Machine Learning Algorithms into Bioinformatics Tools for Enhanced Peptide Identification Accuracy
Machine learning algorithms have been integrated into bioinformatics tools to enhance peptide identification accuracy by allowing for the development of models that can analyze large, complex datasets and identify patterns or features that may not be easily detected by traditional methods. These algorithms can learn from previous data and adjust their parameters to improve performance over time, resulting in more accurate and efficient peptide identification. By utilizing machine learning techniques, researchers can better predict peptide sequences, improve search algorithms, and enhance overall analysis of mass spectrometry data for a wide range of biological applications.

What new software tools have been developed to efficiently handle large-scale mass spectrometry data for peptide identification?
Several new software tools have been developed to efficiently handle large-scale mass spectrometry data for peptide identification, such as MaxQuant, Proteome Discoverer, Skyline, and OpenMS. These tools utilize advanced algorithms and processing techniques to improve the speed and accuracy of peptide identification, enabling researchers to analyze complex datasets faster and more effectively. Additionally, these tools often incorporate features for data visualization, statistical analysis, and automated workflows to streamline the process of identifying and quantifying peptides in mass spectrometry experiments.
Are there any advancements in de novo sequencing algorithms that have significantly improved the identification of novel peptides?
In recent years, there have been several advancements in de novo sequencing algorithms that have greatly improved the identification of novel peptides. One major development is the incorporation of machine learning techniques, such as deep learning, into de novo sequencing algorithms. These approaches have improved the accuracy and efficiency of peptide identification by allowing algorithms to learn patterns and features from large datasets. Additionally, advancements in mass spectrometry technology, such as higher resolution and sensitivity, have also contributed to the improved identification of novel peptides. Overall, these advancements have allowed researchers to more effectively identify and study previously unknown peptides, leading to a better understanding of biological processes and disease mechanisms.
How have database search algorithms evolved to better match experimental MS/MS spectra with peptide sequences?
Database search algorithms have evolved to better match experimental MS/MS spectra with peptide sequences by incorporating advanced scoring methods, improved spectral library searching, and more sophisticated data filtering techniques. These algorithms now utilize probabilistic models, machine learning algorithms, and statistical frameworks to accurately identify the most likely peptide sequences that generated a given spectrum. Additionally, the use of fragment ion information and sequence tags has become more prevalent in matching spectra with peptides, leading to higher confidence in identifying peptide sequences within complex mixtures. Overall, these advancements have greatly enhanced the reliability and accuracy of database search algorithms in matching experimental MS/MS spectra with peptide sequences.
Have there been any breakthroughs in data visualization techniques to aid in the interpretation of mass spectrometry results for peptide identification?
There have been several breakthroughs in data visualization techniques to aid in the interpretation of mass spectrometry results for peptide identification. One such advancement is the development of software tools that can generate interactive visualizations of mass spectrometry data, allowing researchers to easily explore and analyze complex datasets. Additionally, machine learning algorithms have been utilized to create predictive models that can help identify peptides more accurately and efficiently. These advancements have greatly improved the speed and accuracy of peptide identification in mass spectrometry experiments, leading to more reliable results and enabling researchers to gain deeper insights into complex biological systems.
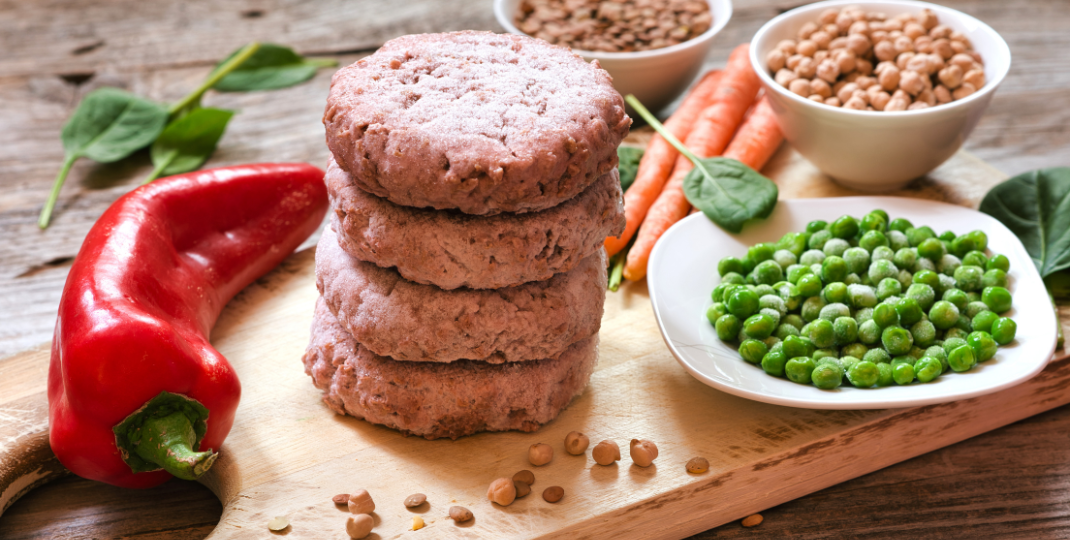
What role do cloud computing and big data analytics play in improving the speed and accuracy of peptide identification by mass spectrometry?
Cloud computing and big data analytics play a crucial role in improving the speed and accuracy of peptide identification by mass spectrometry by providing scalable computing power and advanced data processing capabilities. These technologies enable researchers to efficiently analyze large volumes of complex data generated from mass spectrometry experiments, leading to faster and more accurate identification of peptides. By leveraging cloud-based resources and cutting-edge analytics tools, scientists can streamline the data analysis process, identify potential biomarkers or therapeutic targets, and ultimately accelerate the pace of biomedical research and drug discovery efforts in the field of proteomics.
Have there been any developments in cross-platform compatibility of bioinformatics tools for seamless integration of data from different mass spectrometry instruments?
Yes, there have been recent advancements in improving cross-platform compatibility of bioinformatics tools to facilitate seamless integration of data from various mass spectrometry instruments. These developments include the creation of standardized data formats, such as mzML and mzXML, that allow for the easy exchange and analysis of mass spectrometry data across different platforms. Additionally, there are now software tools and pipelines, such as ProteoWizard and Skyline, that support the processing and analysis of mass spectrometry data generated by different instruments, enabling researchers to compare and combine data from multiple sources more effectively. Overall, these advancements have greatly enhanced the interoperability of bioinformatics tools in the field of mass spectrometry, allowing for more efficient and comprehensive analyses of complex biological samples.
Exploring Efforts to Standardize and Streamline Peptide Identification Workflow Through Unified Software Platforms
Yes, there are ongoing efforts to standardize and streamline the workflow of peptide identification using mass spectrometry through the development of unified software platforms. Several research groups and companies have been working on creating software tools that can efficiently process and analyze mass spectrometry data to identify peptides with higher accuracy and sensitivity. These unified platforms aim to provide a more seamless and standardized approach to peptide identification, allowing researchers to easily compare results across different experiments and laboratories. By consolidating various algorithms and tools into one software platform, these efforts seek to improve the efficiency and reliability of peptide identification workflows in mass spectrometry research.
Advancements in Software Tools and Bioinformatics for Enhanced Peptide Identification by Mass Spectrometry
Advancements in software tools and bioinformatics have greatly enhanced the efficiency and accuracy of peptide identification by mass spectrometry. These advancements include the development of sophisticated algorithms for database searching, improved spectral interpretation methods, and the integration of machine learning techniques for data analysis. Additionally, the use of open-source software and collaborative platforms has facilitated the sharing of knowledge and resources within the scientific community, further driving innovation in the field. Overall, these advancements have significantly accelerated the pace of research in proteomics and have led to a deeper understanding of complex biological systems.
