Data science has emerged as a critical field in the era of big data, machine learning, and artificial intelligence. As businesses strive to make sense of vast amounts of information and uncover valuable insights, startups specializing in data science have become increasingly important. These innovative companies are at the forefront of developing cutting-edge technologies, algorithms, and tools that enable organizations to extract actionable knowledge from complex datasets. In this article, we will explore some of the top data science startups, highlighting their unique contributions to the field and their potential to revolutionize industries across the globe.

What are the top startups in the industry?
There are several top startups in the industry, including Palantir Technologies, which focuses on data integration and analytics for various industries; Databricks, known for its open-source big data processing platform Apache Spark; DataRobot, offering automated machine learning solutions; and Domino Data Lab, providing a collaborative data science platform. These startups have gained recognition for their innovative approaches in leveraging data science techniques to solve complex business problems and drive insights for organizations across different sectors.
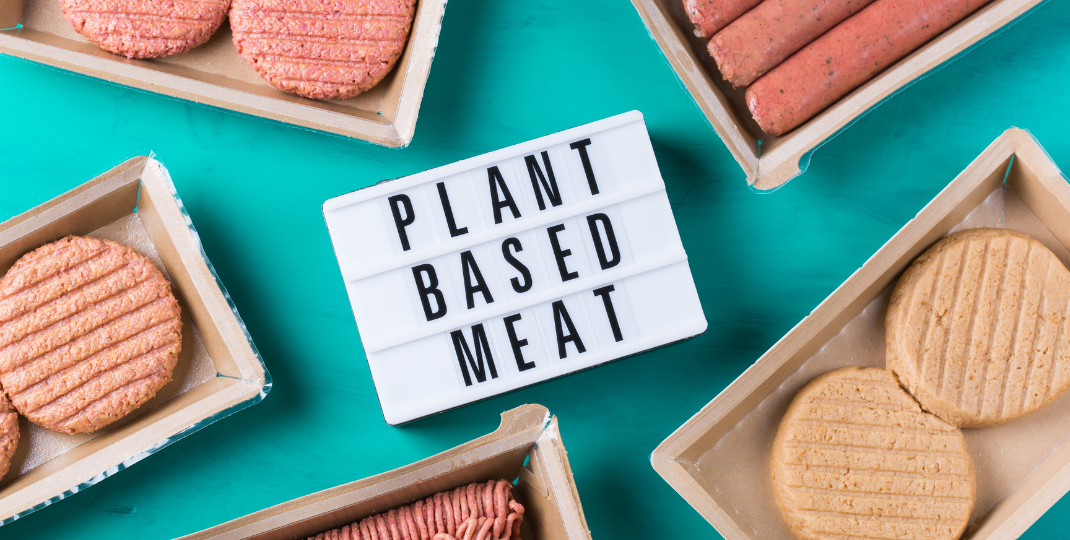
What types of industries do these startups primarily serve?
## How do these startups differentiate themselves from their competitors?
What are the biggest challenges faced by data science startups?
These startups differentiate themselves from their competitors by focusing on unique value propositions and innovative solutions. They often leverage cutting-edge technology, such as artificial intelligence or blockchain, to provide better products or services. Additionally, they may target niche markets or specific customer segments that are underserved by existing players. These startups also prioritize user experience and customer satisfaction, offering personalized and seamless experiences. Furthermore, they strive to disrupt traditional industries by challenging established norms and introducing disruptive business models. Overall, these startups differentiate themselves by being agile, forward-thinking, and constantly pushing the boundaries of what is possible in their respective industries.
How do these startups navigate privacy and security concerns when dealing with sensitive data?
These startups primarily serve a wide range of industries including technology, finance, healthcare, e-commerce, transportation, and energy. They cater to the needs of these industries by offering innovative solutions and services that aim to improve efficiency, productivity, customer experience, and overall business operations. In technology, startups may focus on software development, AI, cybersecurity, or data analytics. In finance, they may offer fintech solutions for payments, lending, or personal finance management. In healthcare, startups may provide telemedicine platforms, health monitoring devices, or digital health records. E-commerce startups may develop online marketplaces or logistics solutions, while transportation startups may focus on ride-sharing or autonomous vehicles. Energy startups may work on renewable energy technologies or energy management systems. Overall, these startups serve various industries with their unique offerings and strive to disrupt traditional practices.
How do data science startups attract and retain top science startups top talent in a competitive job market?
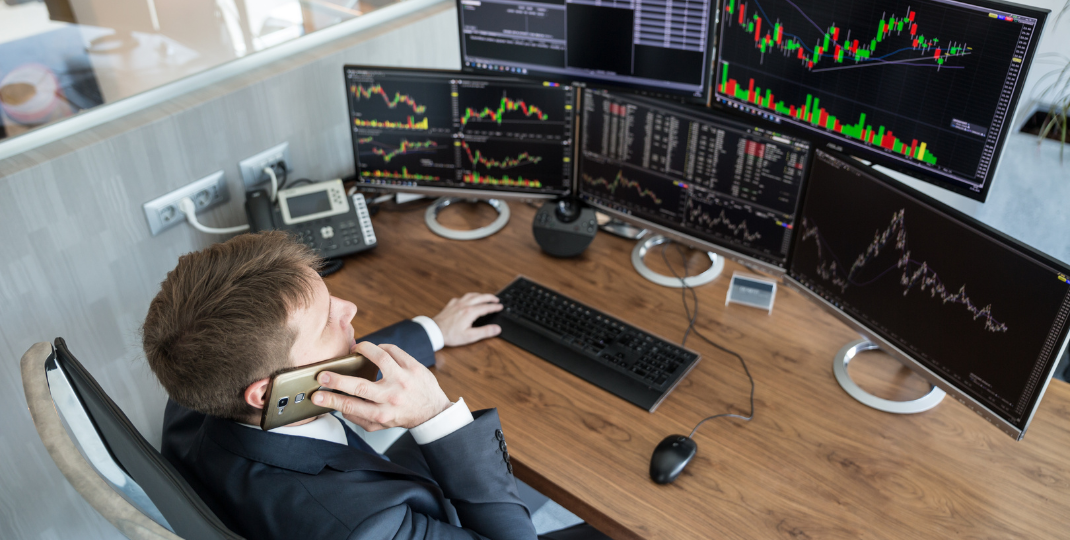
Data science startups face various challenges, but some of the biggest ones include gaining access to high-quality and diverse datasets, building robust and scalable infrastructure for data processing and storage, recruiting and retaining top-notch data scientists and engineers, navigating legal and ethical concerns around data privacy and security, and effectively communicating the value and impact of their data-driven solutions to potential clients or investors. Additionally, competition in the field is fierce, as established tech companies and larger organizations often have more resources and expertise, making it crucial for data science startups to differentiate themselves and continuously innovate to stay ahead.
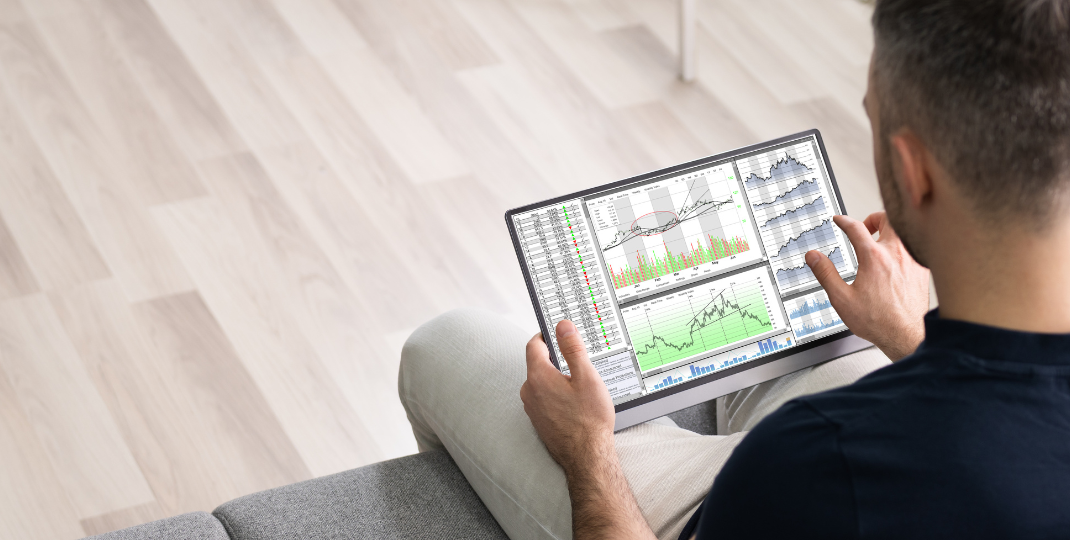
What are the key factors that contribute to the success or failure of data science startups?
Startups navigating privacy and security concerns when dealing with sensitive data typically employ a multi-faceted approach. Firstly, they prioritize data protection by implementing robust encryption techniques, secure data storage practices, and access controls to ensure that only authorized individuals can access the data. Secondly, startups often adhere to strict privacy policies and compliance regulations such as GDPR or HIPAA, which outline the rules governing the collection, use, and storage of personal or sensitive data. Additionally, they may conduct regular security audits and vulnerability assessments to identify and address any potential weaknesses in their systems. Moreover, startups invest in educating their employees about privacy and security best practices to create a culture of data protection awareness. Overall, these measures collectively enable startups to navigate privacy and security concerns effectively and build trust with their customers.
How do these startups approach ethical considerations when developing and deploying data-driven solutions?
Data science startups attract and retain top talent in a competitive job market by offering a combination of attractive incentives and a unique work environment. Firstly, they offer competitive salaries and benefits packages to attract skilled professionals. Additionally, startups provide opportunities for professional growth and learning through training programs, conferences, and collaborations with industry experts. They also emphasize the importance of meaningful work and impact, giving employees the chance to work on innovative projects that have real-world applications. Startups often have a dynamic and collaborative work culture, allowing employees to have autonomy and flexibility in their roles. Furthermore, they encourage a strong sense of community and team bonding through team-building activities and social events. By providing a stimulating and rewarding work environment, data science startups are able to attract and retain top talent in the face of competition.
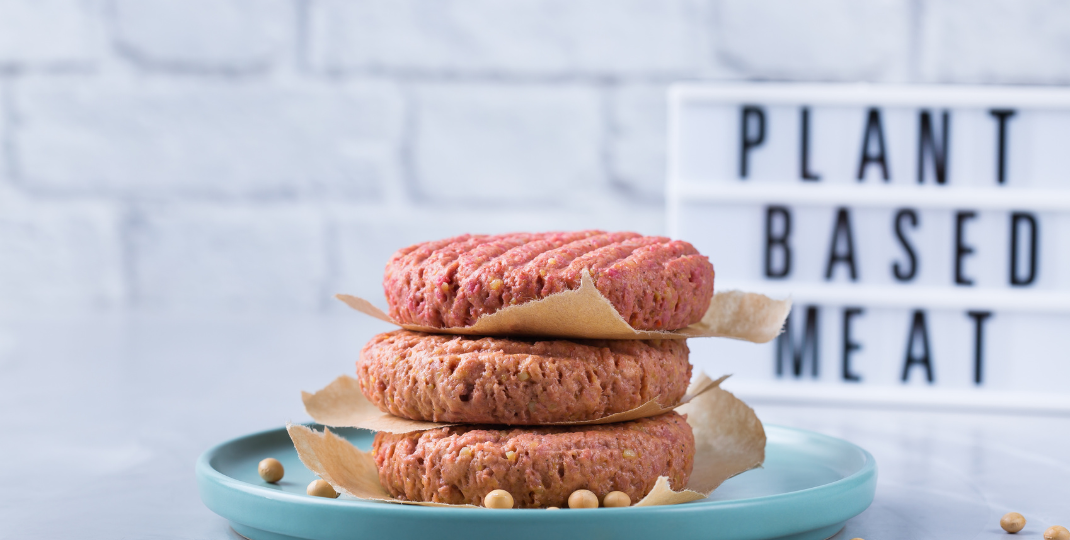
Top Startups: Revolutionizing the Future with Advanced Analytics

The key factors that contribute to the success or failure of data science startups are various and interrelated. Firstly, having a clear value proposition and understanding the problem they aim to solve is crucial. Startups must also possess a team with diverse skills, including technical expertise in data analysis and machine learning, as well as domain knowledge. Securing sufficient funding and having a well-defined business model are vital for sustainability. Additionally, access to high-quality and relevant data, along with the ability to effectively clean, analyze, and derive insights from it, plays a significant role. Lastly, establishing strong partnerships and collaborations, building a scalable infrastructure, and staying updated with technological advancements are essential for long-term success. On the other hand, failure can stem from a lack of market demand, inadequate data quality or quantity, poor execution, insufficient funding, or an inability to adapt to changing market dynamics and evolving technology.
The Benefits of Consuming Enough Mycoprotein for a Balanced Diet
When developing and deploying data-driven solutions, startups approach ethical considerations by emphasizing transparency, accountability, and fairness. They prioritize ensuring that the data they collect and use is obtained legally and ethically, with clear consent from individuals involved. Startups also adopt privacy measures to protect sensitive information and ensure compliance with relevant regulations. They strive to minimize biases in their algorithms and regularly evaluate and audit their models for potential discriminatory impacts. Additionally, many startups engage in responsible data governance and establish guidelines for responsible use, sharing, and disposal of data. They actively seek feedback from their users and incorporate it into their decision-making processes to address any concerns regarding ethical implications. Overall, these startups aim to develop and deploy data-driven solutions that benefit individuals and society while upholding ethical standards.
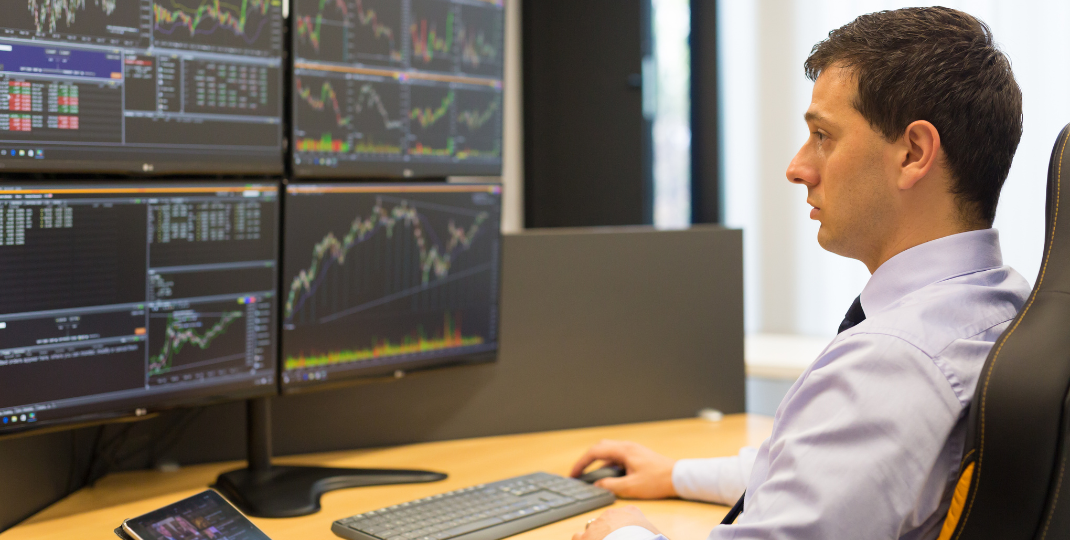
In conclusion, the world of data science has seen a surge in innovative startups that are reshaping industries and pushing the boundaries of what is possible. These top data science startups are leveraging advanced analytics, machine learning, and artificial intelligence to solve complex problems and unlock valuable insights for businesses. With their cutting-edge technologies and expertise, these startups are revolutionizing sectors such as healthcare, finance, e-commerce, and more. As they continue to grow and evolve, it is evident that these data science startups are playing a crucial role in driving digital transformation and shaping the future of our data-driven world.