Startup companies in the field of data science are revolutionizing the way organizations leverage data to gain insights and make informed decisions. With advancements in technology and an abundance of data available, these startups harness the power of algorithms, machine learning, and statistical analysis to extract valuable knowledge from massive datasets. These innovative companies are not only transforming industries but also reshaping the future of business operations by providing customized solutions, predictive analytics, and data-driven strategies. As the demand for data scientists continues to rise, startup companies in this domain are attracting top talent and driving innovation in the rapidly evolving field of data science.

How can startups effectively leverage data science to gain a competitive advantage?
Startups can effectively leverage data science to gain a competitive advantage by utilizing the vast amounts of data available to them. They can analyze customer behavior, market trends, and industry insights to make informed decisions and develop targeted strategies. By employing techniques such as predictive analytics and machine learning, startups can identify patterns and anticipate future trends, enabling them to tailor their products or services to meet customer needs more effectively. Data science also enables startups to optimize their operations by identifying inefficiencies and improving processes. Additionally, startups can use data science to personalize marketing campaigns, enhance customer experience, and drive customer acquisition and retention. Overall, leveraging data science allows startups to make data-driven decisions, stay ahead of the competition, and adapt quickly to changing market dynamics.
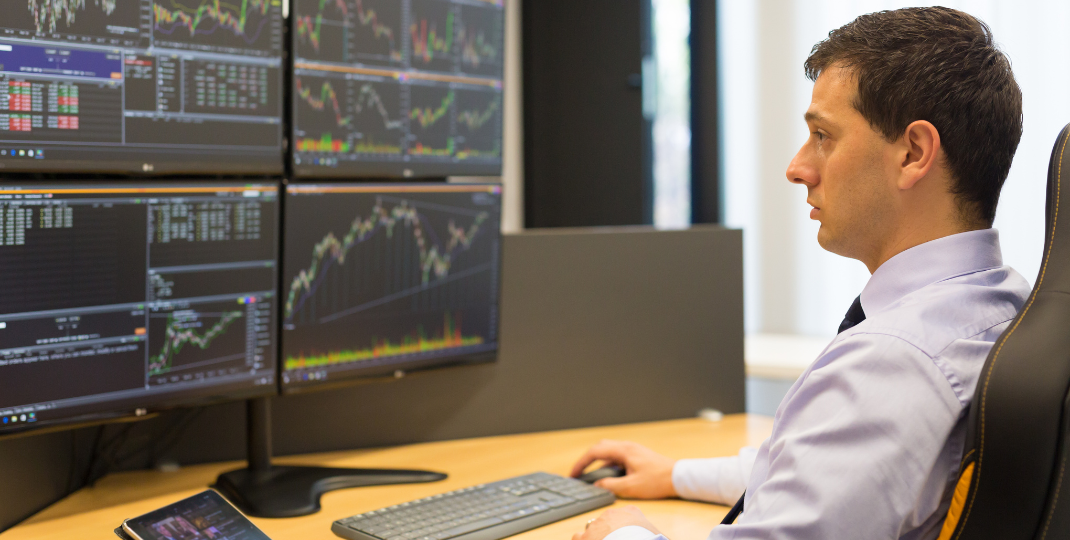
What are the key challenges that startups face when implementing data science solutions?
Startups often face several challenges when implementing data science solutions. Firstly, one major challenge is acquiring and managing the necessary data. Startups may lack access to large volumes of quality data, making it difficult to train accurate models. Additionally, they may struggle with data integration and cleaning processes. Secondly, hiring and retaining skilled data scientists can be challenging for startups due to competition from larger companies and limited resources. Finding individuals with a strong background in both data science and domain expertise can be even more daunting. Lastly, startups often grapple with defining clear business objectives and aligning them with their data science initiatives. It is crucial to identify specific problems that data science can solve and ensure that the solutions are aligned with the startup's overall strategy and goals.
How can startups ensure the accuracy and reliability of their data science models?
Startups can ensure the accuracy and reliability of their data science models by following a few key steps. First, it is important to collect high-quality and relevant data that is specific to the problem being addressed. This involves careful data preprocessing, including cleaning and removing outliers, as well as ensuring data consistency and completeness. Additionally, startups should employ rigorous validation techniques such as cross-validation or hold-out testing to assess the performance and generalizability of their models. Regular monitoring and updating of models based on feedback and new data is also crucial to maintain accuracy. Finally, startups should establish transparency and document their modeling processes, making it easier to replicate and validate the results.
What strategies can startups use to attract top data science talent?
Startups can employ several strategies to attract top data science talent. Firstly, they should establish a strong employer brand by highlighting their unique company culture, mission, and opportunities for growth. Offering competitive compensation packages, including benefits like equity options, can also be attractive to data scientists. Moreover, startups should actively engage with the data science community through networking events, conferences, and online platforms to build relationships and showcase their expertise. Creating challenging and impactful projects, providing access to cutting-edge technologies, and fostering a collaborative work environment can further appeal to top talent in the field. Additionally, promoting a flexible and remote work environment can be advantageous for attracting data scientists who prioritize work-life balance.
How can startups overcome limited resources and budget constraints to invest in data science initiatives?
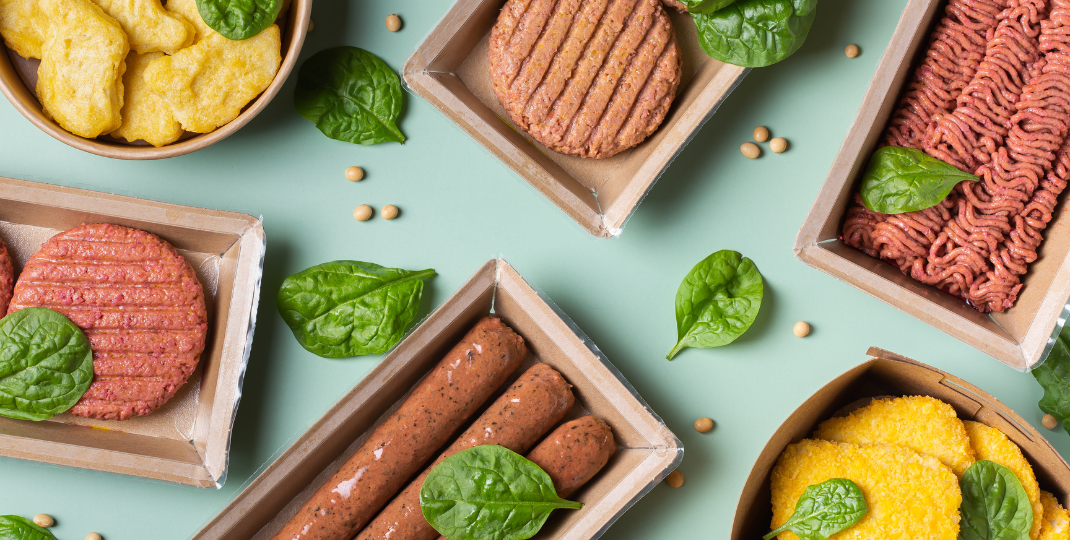
Startups can overcome limited resources and budget constraints to invest in data science initiatives by adopting a strategic approach. Firstly, they can prioritize their data needs and identify the most critical areas where data science can have the highest impact on their business. This allows them to focus their resources efficiently. Secondly, startups can leverage open-source tools and platforms that offer cost-effective solutions for data collection, storage, and analysis. Additionally, they can startup companies for data science explore partnerships or collaborations with universities, research institutions, or established companies to access expertise and resources without significant financial commitments. Startups can also consider hiring freelance data scientists or interns who are eager to gain experience and may work at a lower cost. Lastly, startups can gradually scale their data science initiatives as they grow and generate more revenue, allowing for increased investment in building a robust data infrastructure.
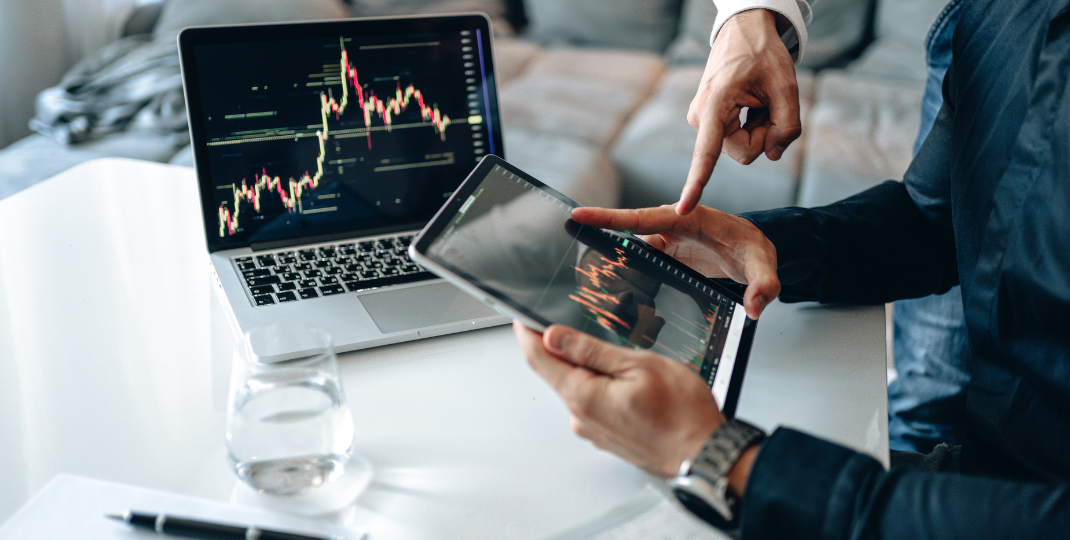
What ethical considerations should startups take into account when collecting and analyzing data?
When collecting and analyzing data, startups should consider various ethical considerations. Firstly, they should prioritize the protection of user privacy and ensure proper consent is obtained before collecting any personal information. Startups should also be transparent about how the collected data will be used and provide users with the option to opt out if they wish. Additionally, startups should ensure that the data they collect and analyze is accurate and reliable, avoiding bias or discrimination in their algorithms or decision-making processes. It is crucial for startups to handle data securely, protecting it from breaches or unauthorized access. Lastly, startups should comply with legal and regulatory frameworks regarding data collection and analysis, such as complying with data protection laws like GDPR or HIPAA. By considering these ethical considerations, startups can build trust with their users and contribute to a responsible and sustainable data-driven ecosystem.
How can startups measure the impact and success of their data science projects?
Startups can measure the impact and success of their data science projects through several key metrics. First, they can track the increase in revenue or cost savings generated directly as a result of implementing data-driven insights. This can be measured by comparing financial performance before and after the project implementation. Additionally, startups can measure the improvement in operational efficiency by monitoring metrics like reduced processing time, increased productivity, or decreased error rates. Another important aspect is customer satisfaction, which can be assessed through feedback, surveys, or customer retention rates. Startups should also evaluate how well their data science projects align with the company's overall goals and strategic objectives. Regular monitoring, tracking, and iteration are essential to continuously improve and optimize data science projects.

What are the potential risks and pitfalls that startups should be aware of when adopting data science technologies?
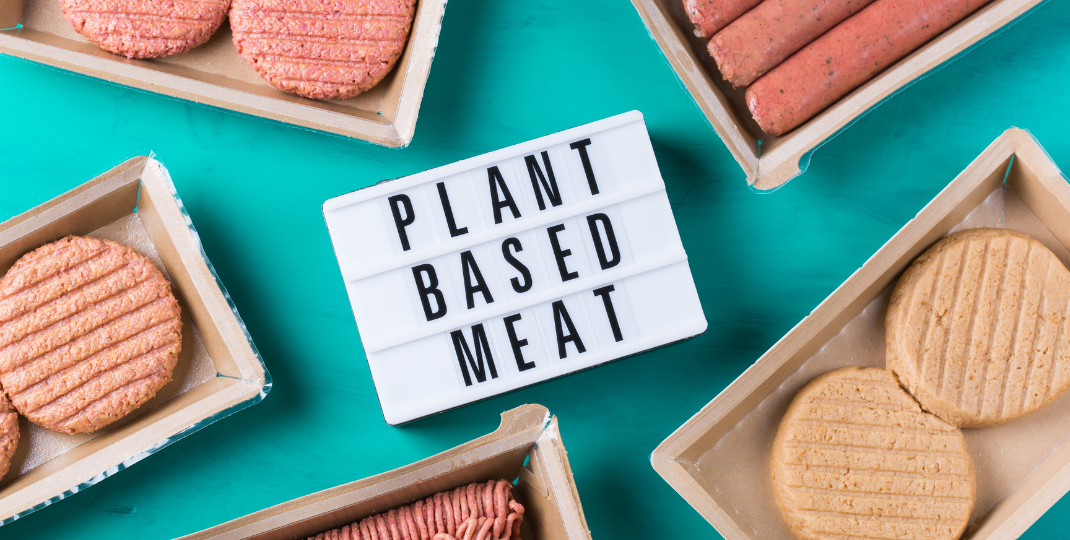
When startups adopt data science technologies, they should be aware of potential risks and pitfalls. Firstly, there is a risk of over-reliance on algorithms and automated decision-making, which may lead to biased outcomes or unintended consequences. Startups should carefully consider the ethical implications and ensure that human oversight is maintained throughout the process. Secondly, data security and privacy are major concerns as startups deal with sensitive customer information. Adequate measures need to be taken to protect data from breaches and ensure compliance with regulations like GDPR. Moreover, the availability and quality of data can pose challenges, as startups might face issues in accessing relevant data or dealing with incomplete or inaccurate data. Lastly, there can be a lack of expertise and skill gaps within the startup team, especially when it comes to interpreting and implementing data science insights effectively. It is crucial for startups to invest in talent development and form cross-functional teams to mitigate these risks and extract maximum value from data science technologies.
The Future of Data Science: Exploring the Promising Landscape of Startup Companies
In conclusion, startup companies in the field of data science have the potential to revolutionize industries and reshape the way we approach problem-solving. With access to vast amounts of data and advancements in technology, these startups are able to provide innovative solutions and insights that were previously unimaginable. They have the agility and flexibility to adapt quickly to changing market needs, making them well-positioned to tackle complex challenges and drive meaningful change. However, it is important for these startups to prioritize ethics and privacy concerns as they navigate the ever-evolving landscape of data science. With proper strategies and a commitment to transparency and responsible data usage, startup companies in data science can pave the way for a future where data-driven decision-making is the norm, benefiting businesses and society as a whole.
