Database search algorithms play a crucial role in peptide identification by mass spectrometry, as they are essential for matching experimental mass spectra to theoretical spectra generated from protein sequence databases. By comparing the fragmentation patterns of peptides generated during mass spectrometry to those stored in databases, these algorithms can accurately identify and characterize proteins present in a sample. This process allows researchers to quickly and efficiently analyze complex proteomic data, leading to a deeper understanding of biological systems and disease processes. In this way, database search algorithms serve as a powerful tool for advancing our knowledge of the proteome and identifying potential biomarkers for various health conditions.
Understanding the database search algorithm for identifying matching peptides using mass spectrometry data
The database search algorithm for matching peptides based on mass spectrometry data typically involves comparing the experimental peptide masses obtained from the mass spectrometer with theoretical peptide masses generated from a protein sequence database. The algorithm calculates the mass-to-charge ratio of each peptide ion and searches the database for peptides that have similar or identical masses within a specified tolerance. It then scores the matches based on factors such as the number of matched peaks, intensity, and fragmentation pattern. The algorithm ranks the identified peptides by their scores and reports the top matches as potential peptide identifications.
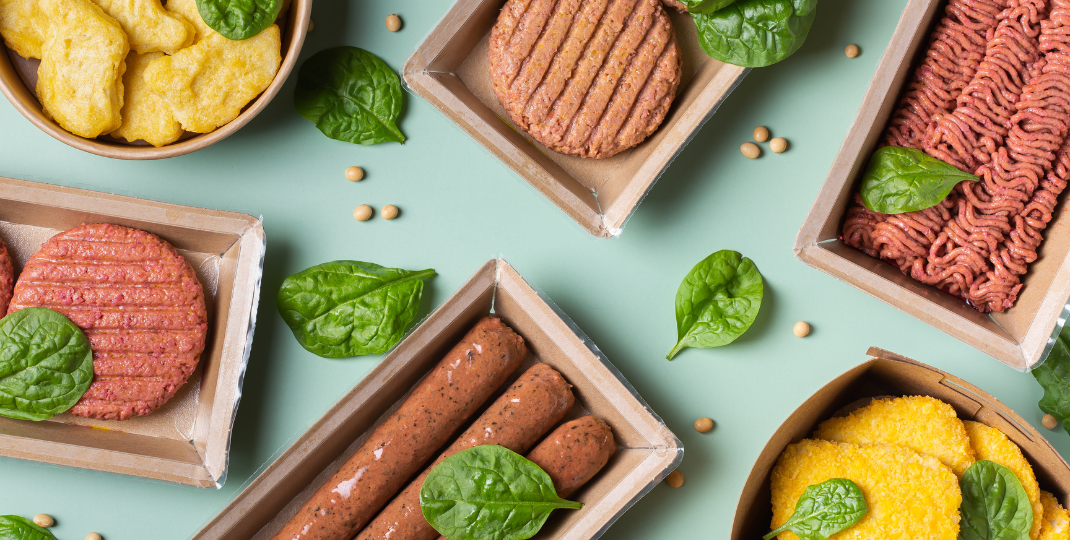
What factors influence the accuracy and efficiency of database search algorithms in peptide identification?
The accuracy and efficiency of database search algorithms in peptide identification are influenced by a variety of factors. These factors include the size and quality of the database being searched, the scoring system used by the algorithm to rank potential matches, the sensitivity and specificity of the search parameters set by the user, and the computational resources available for running the algorithm. Additionally, factors such as the complexity of the sample being analyzed, the presence of post-translational modifications, and the level of noise in the data can also impact the accuracy and efficiency of the algorithm. Overall, a combination of these factors must be taken into consideration when designing or selecting a database search algorithm for peptide identification in order to achieve optimal results.
How do database search algorithms differentiate between true peptide identifications and false positives?
Database search algorithms differentiate between true peptide identifications and false positives by using a scoring system that evaluates the match between the observed mass spectrum data and theoretical spectra generated from the protein sequence database. These algorithms consider factors such as the number of matched peaks, the intensity of the peaks, and the presence of unique peptides or modifications. Additionally, statistical methods such as calculating the false discovery rate (FDR) are used to estimate the probability of a given identification being a false positive. By incorporating these criteria and statistical analyses, database search algorithms can effectively distinguish between true peptide identifications and false positives.
Are there different types of database search algorithms used in peptide identification by mass spectrometry?
Yes, there are different types of database search algorithms used in peptide identification by mass spectrometry. Some of the most commonly used algorithms include SEQUEST, Mascot, X!Tandem, and Comet. These algorithms work by comparing the experimental mass spectrometry data of peptides with theoretical spectra generated from protein sequence databases to identify and match peptides. Each algorithm has its own unique scoring system and method for determining the best match between experimental and theoretical spectra, ultimately leading to the identification of peptides in complex mixtures.
How do database search algorithms handle post-translational modifications and sequence variations in peptide identification?
Database search algorithms handle post-translational modifications and sequence variations in peptide identification by comparing experimental data (such as mass spectrometry results) with theoretical peptide sequences generated from a protein database. These algorithms consider various modifications that can occur on peptides, such as phosphorylation, glycosylation, and acetylation, by incorporating them into the search parameters. Additionally, they account for sequence variations due to single nucleotide polymorphisms or alternative splicing events by allowing for mismatches or gaps in the alignment between experimental and theoretical peptide sequences. By considering these modifications and variations, database search algorithms improve the accuracy of peptide identification in complex biological samples.

What are the limitations or challenges faced by database search algorithms in peptide identification?
Database search algorithms for peptide identification face several limitations and challenges, including the vast size and complexity of protein databases, leading to longer search times and increased computational resources. Additionally, the presence of post-translational modifications, sequence variants, and database redundancy can hinder accurate peptide identification. Moreover, incomplete or incorrect protein annotations in databases can result in false positive identifications. The high sensitivity and specificity required for reliable peptide identification also pose a challenge, as algorithms must balance between minimizing false positives and false negatives. Overall, the constantly evolving nature of protein databases and the complexity of biological samples make it difficult for database search algorithms to achieve optimal performance in peptide identification.
How do database search algorithms deal with large and complex proteomic datasets in peptide identification?
Database search algorithms use indexing techniques to efficiently search large and complex proteomic datasets for peptide identification. These algorithms break down the dataset into smaller, more manageable chunks and create indexes based on specific criteria such as amino acid sequences or mass-to-charge ratios. By using these indexes, the algorithms can quickly narrow down the search space and identify potential peptide matches. Additionally, algorithms may utilize advanced scoring methods and statistical analysis to accurately match peptides to experimental data and reduce false positives. Overall, database search algorithms help researchers effectively navigate vast proteomic datasets and extract meaningful information for further analysis and interpretation.
What advancements or improvements have been made in database search algorithms for better peptide identification accuracy and sensitivity?
Advancements in database search algorithms for peptide identification have focused on improving both accuracy and sensitivity. One major improvement has been the development of more sophisticated scoring systems that take into account a variety of factors such as mass accuracy, peptide length, fragmentation patterns, and post-translational modifications. Additionally, machine learning techniques have been employed to help the algorithms better distinguish between true peptide identifications and false positives. Other improvements include the use of decoy databases to estimate false discovery rates, as well as the integration of multiple search engines to increase overall confidence in peptide identifications. These advancements have led to significant improvements in the accuracy and sensitivity of peptide identification in proteomics research.
The Crucial Role of Database Search Algorithms in Peptide Identification by Mass Spectrometry
Overall, database search algorithms play a crucial role in peptide identification by mass spectrometry. These algorithms help to quickly and accurately match the experimental data with theoretical peptide sequences stored in large databases, allowing researchers to confidently identify proteins and gain valuable insights into biological processes. By efficiently sorting through vast amounts of data and identifying potential matches, database search algorithms streamline the process of peptide identification, ultimately advancing our understanding of complex biological systems and accelerating scientific discoveries.
